- AI Enterprise Vision
- Posts
- Data: The New Oil Crisis for AI?
Data: The New Oil Crisis for AI?
Data Deluge or Drought?
We've been chugging along, belching out data left, right, and center - feeling pretty smug about it.
But here's the twist folks: what if our tank's running dry?
Sit tight, because we're about to take a hard look at the world of training datasets and the twilight zone of unlabeled data.
The scary part?
We might just be running on fumes.
[The entire content of the article is attributed to the wonderful paper provided in the reference]
Riding the Data Growth Express: Choo-choo!

Our training datasets for vision and language models are ballooning faster than a politician's promises pre-election.
But are we signing checks our data banks can't cash?
With AI's appetite for data, we might be barreling towards a 'sold out' sign, and soon!
The Great Language Data Tsunami

Here's a fact to make your head spin.
We've stockpiled language data up to a dizzying 2e12 words.
But brace yourselves - the tide might be turning.
As the data surge starts to ebb between 2030 to 2050, AI's going to find its language capabilities high and dry.
Crunch Time for High-Quality Data
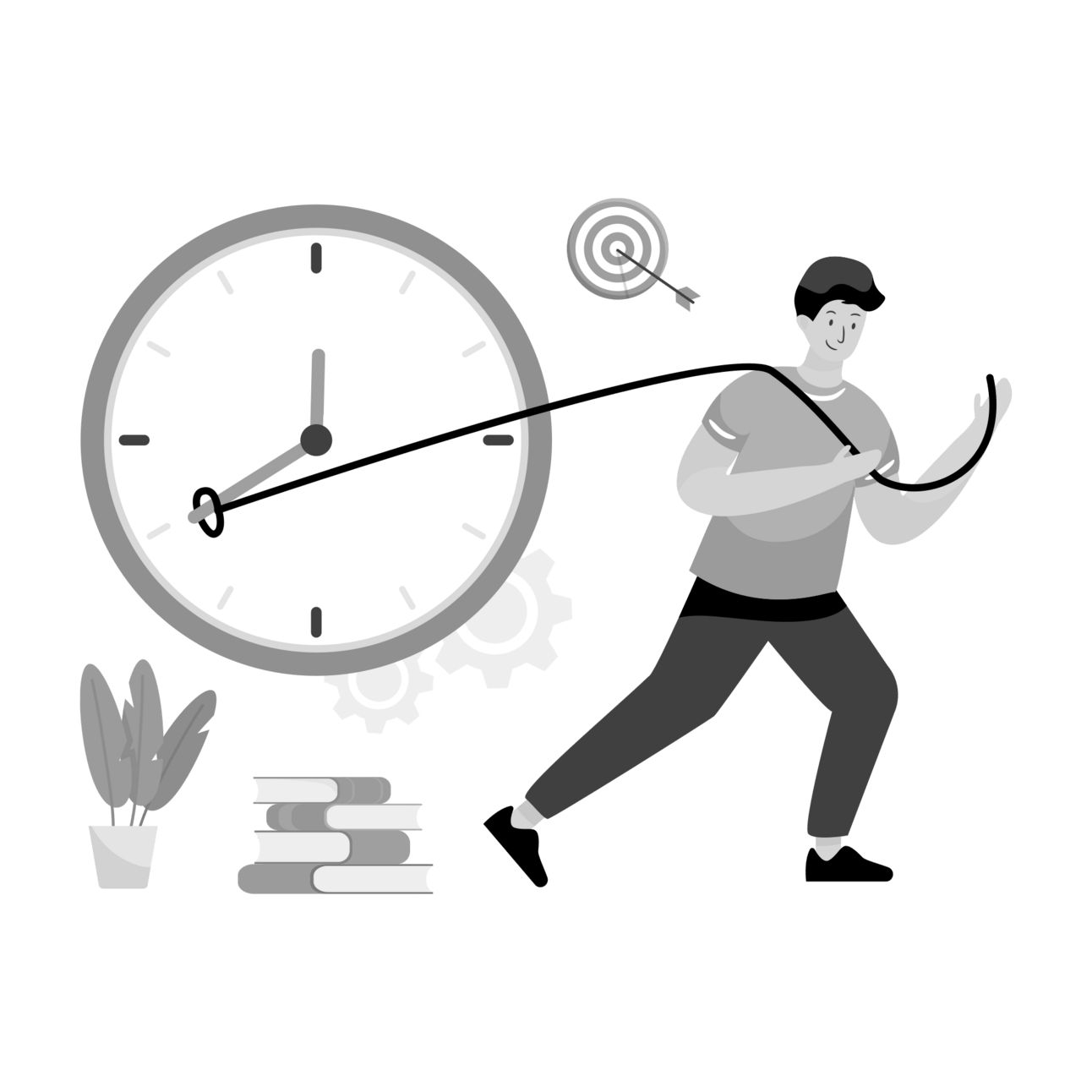
It's not just about filling AI's belly - it's about giving it a gourmet experience. AI feasts on curated datasets like a food critic at a 5-star restaurant.
But even this buffet is starting to look sparse.
The next course?
A dry spell in high-quality data between 2023 and 2027. Now there's a bitter pill to swallow.
The Vision Data Predicament

We've all heard the saying, "A picture is worth a thousand words," but what happens when the pictures run out?
Our AI could hit a blind spot between 2030 and 2070.
Without the right visuals on the menu, AI's feast turns into a fast.
Hitting the Data Bottleneck

Here's the grand reveal: we're zooming straight towards a data traffic jam.
AI is guzzling data faster than we can pump it.
If we can't fill the tank, we're going to be stranded on the AI highway, folks.
Not the best ending to our joyride, is it?
A Pinch of Salt: Weighing the Caveats

Now, before we all pack up and head for the hills, let's pump the brakes a bit.
We've thrown a lot of numbers and predictions your way, but it's only fair we air out the laundry, too.
Here are some potholes that might throw off our estimates:
Today's Data Guzzler, Tomorrow's Hybrid: AI might become more efficient and need less data for top performance. History's shown us this could be a real possibility.
Compute Availability Hitting a Speed Bump: Our computing power could grow slower than expected due to pesky tech issues, supply chain snags, or maybe we just don't want to break the bank.
The Map's Not the Territory: Our current scaling laws could be taking us on a wild goose chase. Who knows, we might discover more efficient scaling routes that are less data-hungry.
It's a Mixed Bag: Multimodal models might outperform single-modality ones via transfer learning, effectively turning our data stock into a sumptuous smorgasbord.
There are also a few "maybes" when we talk about our data stock estimates:
The Synthetic Goldmine: Synthetic data could make our data stock infinite. But the jury's still out on its cost-effectiveness and usefulness in training.
Riding the Economic Wave: Big economic shifts, like widespread adoption of self-driving cars, could open the data floodgates. Imagine all that road video data!
Big Budget, Big Data: Governments or large corporations might play sugar daddy and increase data production with enough dough. Think screen recording and mass surveillance on steroids.
Alchemy: Converting Low into High: We might find ways to spin straw into gold, or rather, low-quality data into high-quality data, through robust automatic quality metrics.
Remember, folks, we're working with estimates here.
These caveats are not to toss cold water on your data worries but to ensure we're not getting carried away on a one-way track.
After all, it's not just about reaching the destination but also enjoying the ride!
Running on Empty? The Solution

So, what's our game plan?
We need to push the pedal to the metal and rev up our data engines. It's time to get creative, broaden our horizons, and rally the troops.
We've got to stay in the race, making sure we're considering the ethical pit stops along the way.
Because, let's face it - nobody wants to be disqualified from the AI Grand Prix.
So there it is, the data dilemma laid bare.
We've been cruising along, riding high on our data production.
But the road ahead might be steeper than we thought. If we don't want our AI journey to grind to a halt, it's time to kick things into high gear.
Because the end goal is a future where AI is breaking records, reshaping the world, and improving our lives. So let's roll up our sleeves and keep the tank full, folks.
Onwards and upwards!
References
[1] The entire content of the article is attribute to the wonderful paper written here —-Will we run out of data? An analysis of the limits of scaling datasets in Machine Learning