- AI Enterprise Vision
- Posts
- Human-Centric AI: The Diverse Skill Set AI Truly Requires
Human-Centric AI: The Diverse Skill Set AI Truly Requires
The AI Talent Discussion Should Look Beyond Just Technical Skills
The growing conversation around an AI talent shortage often focuses narrowly on data science and engineering capabilities.
While foundational, this risks limiting the scope of what meaningful AI talent represents in a holistic sense.
Successfully navigating the AI era requires diversity in skillsets that spans technology, human sciences, ethics, and strategy.
AI talent shortages absolutely exist within core technical domains, and these gaps need addressing to boost capabilities.
However, equally important are non-technical roles that complement the hard skills:
AI Strategists

AI Strategists who synthesize business needs, data dynamics, and ethical risks to craft holistic AI roadmaps.
Responsibilities:
Analyze business objectives and pain points to determine where AI could provide value
Conduct feasibility studies on proposed AI solutions considering data, infrastructure, and algorithm limitations
Develop comprehensive AI strategies and roadmaps balancing business impact, technical complexity, and ethical considerations
Create frameworks to monitor and measure ROI, risks, and KPIs across AI projects
Continuously align AI initiatives with evolving business goals and capabilities
Questions they address:
What are the highest value AI opportunities aligned to business goals?
Is pursuing a proposed AI solution truly feasible given available data, infrastructure, and algorithms?
How do we ensure AI projects deliver maximum benefit with minimal risk?
How can we iteratively prioritize AI investments as business needs change?
Behavior Scientists

Guide human-centric AI by understanding human cognition, emotion, motivations, and biases. How will people interact with and be impacted by AI?
Responsibilities:
Deeply study target users through ethnographic research, interviews, surveys, and psychological assessments
Identify motivations, heuristics, biases, pain points, and needs driving user behavior
Guide data collection, labelling, and testing methodology to capture diverse user perspectives
Ensure AI systems align with nuances in human cognition, emotion, culture, and moral values
Continuously assess user experience and feedback to refine AI human-centricity
Questions they address:
How exactly will different types of users perceive, interact with, and be impacted by an AI system?
What unintended consequences could emerge from mismatches between AI and human cognition/values?
How do we collect unbiased, representative data on diverse user populations?
How can we systematically measure and improve human-AI compatibility?
AI Ethicists

Ensure alignment with human values by examining philosophical and societal impacts of AI. What are the ethical boundaries we must operate within?
Responsibilities:
Lead ethics reviews spanning data collection, algorithm design, applications, and impact
Develop organizational frameworks, policies, and governance for ethical AI development
Advocate policies that protect broader society from AI harms like bias and misinformation
Educate developers and users on AI ethics to promote accountability and transparency
Continuously monitor AI systems and usage for potential issues like privacy invasion
Questions they address:
Does an AI system undermine or align with fundamental human values?
Could the AI directly or indirectly cause harm at individual or societal levels?
How do we ensure ethical AI practices while retaining innovation and impact?
How can we maximize benefits of AI while proactively mitigating risks?
How should organizations be held accountable if AI systems produce harmful outcomes?
AI Adoption Experts

Enable integration of AI through change management, communication and training. How do we successfully incorporate AI capabilities?
Responsibilities:
Conduct change management activities to ready organization for AI integration
Develop communication strategies to build AI understanding and buy-in at all levels
Design and deliver AI training programs tailored to diverse audiences and roles
Consult on user experience design and workflows to optimize human-AI collaboration
Continuously gather user feedback to refine adoption and minimize backlash
Questions they address:
How do we motivate people across the organization to embrace AI-driven change?
What customized education does each role need to successfully adopt AI?
How should responsibilities between humans and AI be designed for maximum synergy?
What adaptations maximize user adoption, utilization, and satisfaction of AI tools?
How can we systematically gather user feedback to refine and expand adoption?
Multidisciplinary AI Generalists
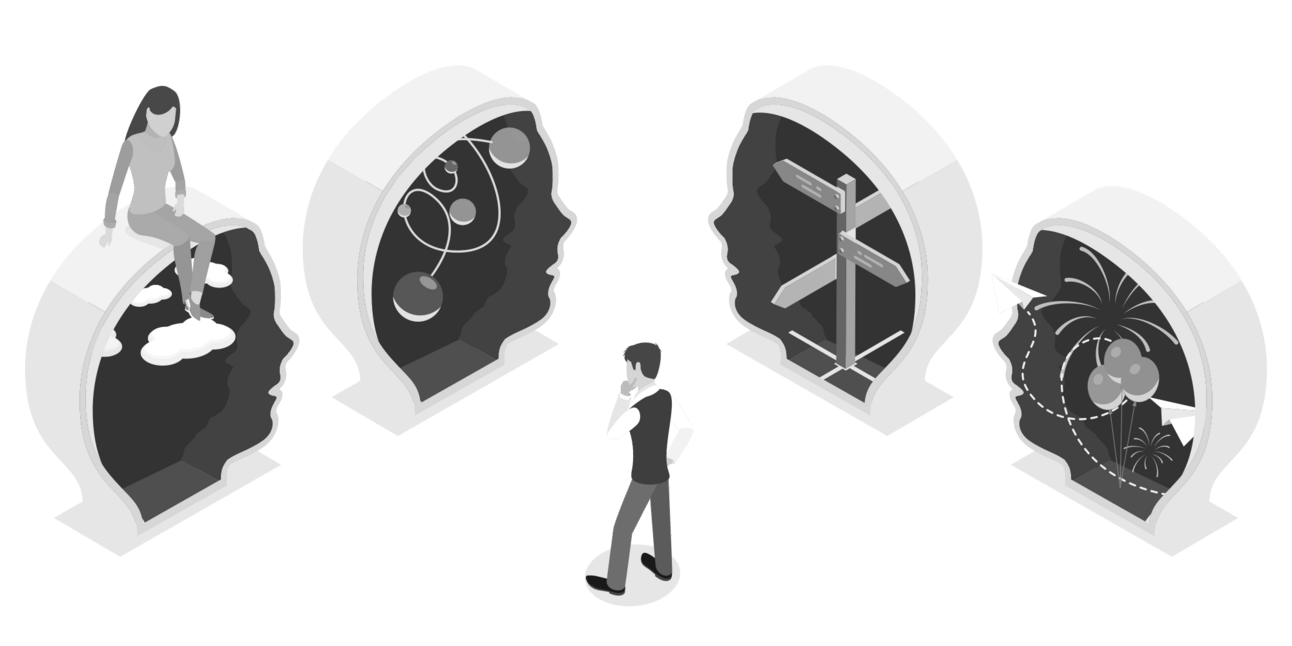
Translate business challenges into AI solutions by bridging technical and non-technical domains. What AI approaches can address real-world problems?
Responsibilities:
Formulate AI solutions by synthesizing inputs across business, technology, human, and ethics domains
Rapidly prototype and test AI concepts to determine feasibility and refine requirements
Communicate complex AI approaches and implications to diverse audiences
Guide implementation by coordinating complementary roles like data scientists, engineers, designers, and subject matter experts
Continuously expand T-shaped capabilities spanning technical AI expertise and interdisciplinary soft skills
Questions they address:
What hybrid approaches combining AI with other solutions can address the problem?
How do we distill complex real-world problems into appropriate AI solutions?
What are the dependencies and risks across domains like human behavior, data, algorithms, and business processes?
How can we translate AI concepts into concrete capabilities that solve real user needs?
What combination of multidisciplinary skills are needed to successfully deliver AI solutions?
Discussions of "AI talent" must expand beyond coding and data science.
Enterprises need talent diversity spanning technology, human sciences, creativity and ethics to deliver responsible and impactful AI adoption.
This means rethinking talent strategies - sourcing more non-technical domain experts and fostering collaboration and cognitive diversity in AI teams.
The combination of left-brain technologists and right-brain human specialists will define the pioneers in human-centric AI.