- AI Enterprise Vision
- Posts
- Let's Understand: How the 'Attention' Trick Helps Computers Talk Like Us!
Let's Understand: How the 'Attention' Trick Helps Computers Talk Like Us!
AI Public Literacy Series- ChatGPT Primer Part 3c
Ever wondered how ChatGPT or Claude can chat with you like a human pal, understanding what you say and responding sensibly?
This magic is brought to life by a clever little trick known as the attention mechanism.
Join us as we uncover this secret and see how it helps language models to understand and generate human-like responses.
The Attention Spotlight

Think about a stage play.
You know how a spotlight follows the actors around to highlight who's the most important at that moment?
The attention mechanism does the same thing with words in a sentence.
It shines a metaphorical spotlight on words, showing how important they are in the grand scheme of the conversation.
Putting Weights on Words

When the spotlight falls on a word, the attention mechanism gives it a 'weight', which is a measure of its importance.
It's just like in a play where the main actors get more time in the spotlight because their roles are crucial.
Similarly, the attention mechanism assigns more 'weight' to the important words.
Unraveling Word Connections

Imagine a story where everything's connected across different chapters.
What happens in the last chapter often depends on what happened way back in the first few.
The attention mechanism helps language models to follow these long-range connections.
Just like a reader picks up on connections between different parts of a story, the attention mechanism assigns weights to words, understanding their relationships.
This makes it possible for the model to understand the broader context, just like a good reader does.
Understanding Language Better
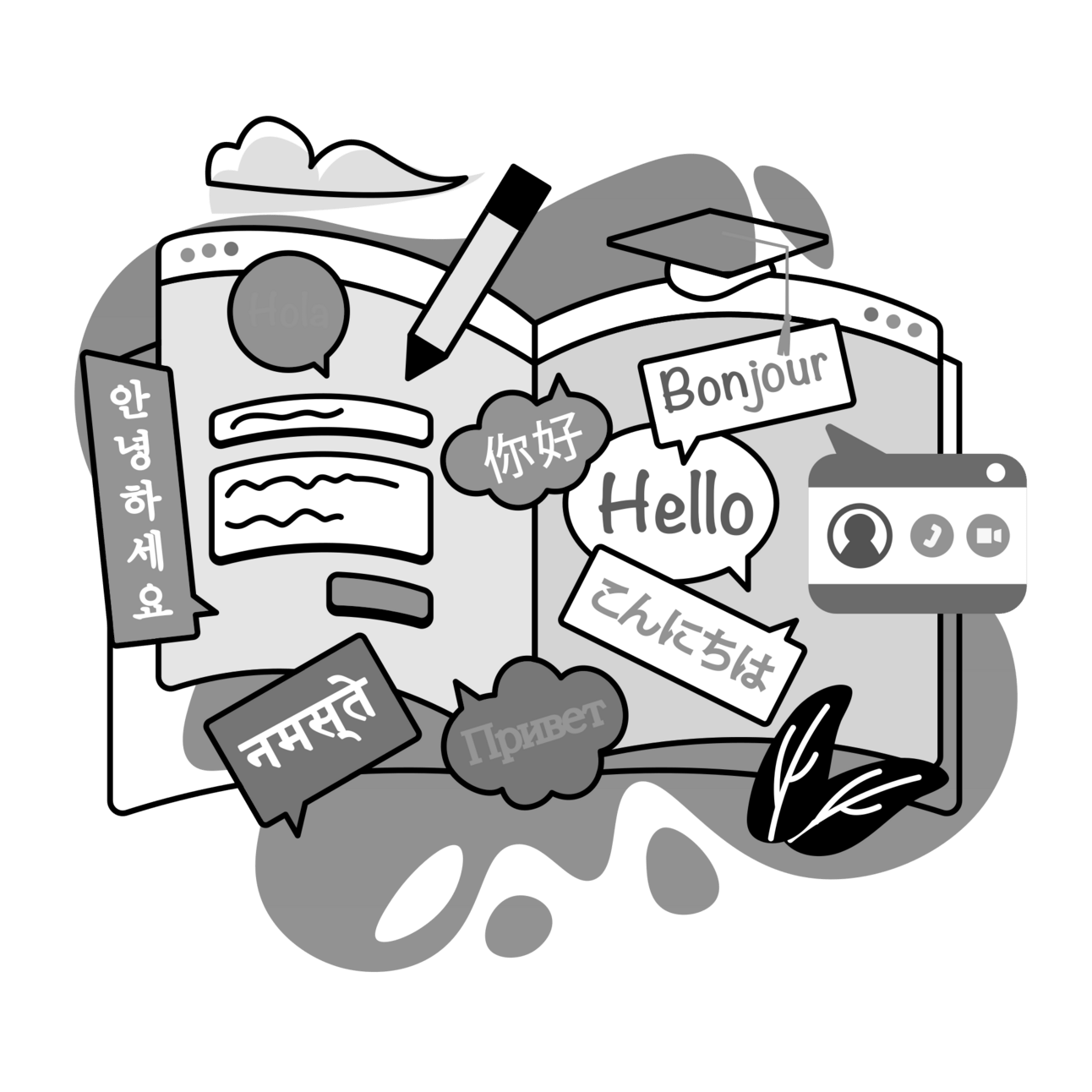
Thanks to the attention mechanism, language models get better at understanding the complexities of language.
It helps them get the overall meaning, connections between words, and the subtle nuances of language.
For instance, in a sentence like "The sun shines brightly in the sky", the attention mechanism could put more weight on "sun" and "shines", highlighting their significant connection.
This understanding lets the model generate responses that are contextually accurate and make sense.
Throwback: The Old Way of Sequential Processing

Before the attention mechanism came along, language models would process words one after the other in order, known as sequential processing.
But this didn't capture long-range connections between words too well.
Enter attention mechanism, the game-changer.
The Present and the Future

Today, attention has become a critical part of modern language models.
Think GPT-3, BERT, and T5 - they all rely on attention to understand and generate language accurately.
Looking ahead, researchers have been playing around with different kinds of attention mechanisms, like multi-head attention or sparse attention.
These variations help models look at different aspects of the conversation, understanding a wider range of connections.
At the same time, people are working on combining attention with other techniques like reinforcement learning and unsupervised learning.
This could lead to even better understanding of language.
Wrapping Up
The attention mechanism works like a guiding spotlight that helps language models understand and generate language accurately.
By assigning weights to words and capturing connections between them, it enhances language understanding.
From freeing language models from the limitations of sequential processing, to powering today's advanced models, the attention mechanism has come a long way.
As we move into the future, it's exciting to see how attention will evolve, with ongoing research exploring different attention mechanisms and their integration with other learning techniques.
As attention mechanisms become more sophisticated, we can look forward to language models that are capable of more complex and human-like interactions.
So the next time you're amazed at how well ChatGPT or Claude understands you, spare a thought for the attention mechanism working behind the scenes.